The study of causality has fascinated humans since the pre-Socratic ages when Democritus had supposedly confessed: “I would rather discover one cause than gain the kingdom of Persia.”
Needless to say, he didn’t do too well with either, and it’s widely believed that Plato was so miffed at his ideas that he wished all of his books were destroyed. Centuries hence, we remain in love with the notion of causality and ponder over fundamental questions about human behaviour and the world around us. While the ancient Greeks had an uncanny knack for asking (and often just knowing the answers to) foundational questions about humans and their relationship with the external world, what they couldn’t have predicted, with all their collective wisdom, was that someday we would systematically cultivate and exploit data, leading up to this data revolution that we are currently witnessing.
This deluge of “big data” generated each day through numerous activities in the real world or on the Internet, contribute to one of the largest sources of information about social behaviour today. Consider, for instance, how Facebook currently has over 1.7 billion monthly active users who collectively generate over 4 new petabytes of data each day. A massive 4 million likes are produced on the site every minute, and till date 250 billion photos have been uploaded to the platform. That is a lot of data, and we are only just talking about a single platform on the vast Internet. Welcome to the data generation!

Back to causality, one tends to wonder what implications this fast-food-like availability and sheer scale of data might have towards the way we study questions around causality. Now, while I would have loved to do an entire post on the essence of causality, its philosophical underpinnings, and the many competing schools of thought on deriving “causal” estimates, I must leave that for a later post. What I would recommend, however, would be to read up on work by Donald Rubin and Judea Pearl, two giants in the field who have often been on opposite sides of the causality debate (the key points of which were neatly illustrated in Andrew Gelman’s blog).
In today’s post, I’ll steer clear of the above debate and focus instead on the role of large observational data in causality research. Through the next half of this post, the key question I hope to pose is about whether, and how we can address causality related questions differently with big data.
A point that needs to be made clear at the very outset when talking about causality and big data is that neither the size of data nor the easy availability serves as a silver bullet. In fact, while there are well-known instances in machine learning , where more data helps scale up model performance (e.g. non parametric methods, deep learning architectures etc.), this doesn’t hold up when searching for causality. In almost every research context I’ve encountered, data tends to play second fiddle to the study design and the presence of a sound theory, that helps anticipate and explain findings. Indeed, statistical assumptions such as the exogeneity of variables, inclusion of pre-treatment and exclusion of post-treatment variables etc. are all equally binding in small or big data contexts. Statistically speaking, the size of the data, be it the number of rows or the number of columns, does not really
have a significant play here. So, to answer the question: Does an automatic and exhaustive “kitchen-sink” approach of applying big data to answer questions around causality work? NO, not in the general case at least.
This is not to suggest, however, that big data has no play in “how” we go about doing causality research. In fact, I would argue that the availability of such large and multidimensional datasets have now made it possible to analyse several new research questions, which were previously considered unapproachable. In a way, I think of big data as a key that has opened up a whole new world in empirical research, purely because of two key affordances : 1. automated hypothesis generation 2. fostering causality research in domains where experimental work is problematic.
First, and every machine learning researcher would attest to the fact that an obvious advantage of having access to large volumes of data is pattern recognition. For social scientists, this translates to analysing big data in search of what can best be described as “empirical regularities” in a particular context. For instance, in my research on the offline impacts of online engagement at a large social network site (SNS), I analysed millions of site usage and transactional logs to find that the offline spending of customers decreases on average whenever they join online brand pages on the SNS. This is an empirical regularity and goes against popular intuition. We then leveraged existing theories and research designs to explain this regularity in our paper. In other instances from fields like the medical sciences, automated data-driven approaches can parallelly test millions of hypotheses on which protein correlates well with a particular disease. While this does not directly answer causal questions, it reduces the set of “potentially causal” hypotheses to a more manageable set, allowing the researcher to then design clinical trials to further investigate the causal linkages.
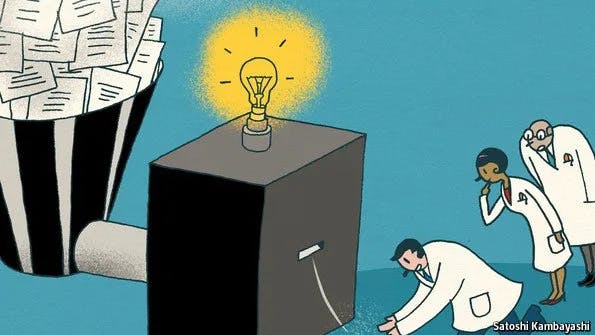
Second, it is easy to think of situations where the use of experimental methods is either impossible or impractical. Let me cite two popular examples here.
For a large part of the 20th century, scientists and policy makers have been debating tobacco companies over whether smoking causes cancer (or not). A 1964 Report on Smoking and Health by the US Surgeons General claimed that cigarette smoking caused a 70% increase in the mortality rate of smokers, over non-smokers. However, and interestingly, the report came under severe criticism from not just tobacco companies, but also leading scientists and statisticians, a position that was held by the great Ronald Fisher too. The main criticism was that the assertions in the report and in several such publications around the time were purely based on correlations, and hence did little to prove causality in any conclusive way.
The empirical problem, however, is in designing an effective randomised experiment. (i.e.) to test the effect of smoking, we would ideally need a treatment group of individuals who are made (persuaded?) to smoke. Quite obviously, this is neither easy nor ethical. The second such example comes from the realm of social network sites (SNS). Think about the problem of conducting experiments on large social networks with potentially millions of observed and unobserved links among the actors. Now think about how you might perform randomised assignments in such networked contexts, such that the users in the treatment group are completely isolated from those in the control group. This is a complicated theoretical problem since actors in a connected graph (e.g. Facebook) are linked to each other through direct or indirect links. This is a problematic situation and a good example of a research context which warrants non-experimental approaches.

Note: Estimated average degrees of separation between all people on Facebook. The average person is connected to every other person by an average of 3.57 steps. The majority of people have an average between 3 and 4 steps.
Popular quasi-experimental techniques include the propensity score matching methods (PSM), instrumental variables, regression discontinuity and natural (quasi-) experiments. Broadly, these approaches leverage the availability of a large number of variables in the dataset to correct for biases introduced by unobservable factors (e.g. user intention or motivations). For instance, PSM models the selection of individuals based on observable factors, and constructs a matched quasi-control group of users who have similar propensities of getting treated (but did not, for whatever reasons). To this effect, the presence of high-dimensional datasets is beneficial in ruling out certain types of biases. However, this does not in any way discount the importance of having a sound theory and design. Nor should we over-rely on large datasets as they are often generated by corporations and individuals with specific motives. Simply put, observable datasets are often far removed from the data generating process, or what computational theorists might call, the Oracle.
Final thoughts – big data has captured the imagination of an entire generation of researchers, but statisticians recommend caution when trying to answer questions that are inherently causal in nature. While a large sample size can be helpful in increasing the precision of estimates or the power of statistical tests, it is not effective at improving the consistency of statistical estimators, nor is it informative about the underlying mechanisms guiding the data generating process. However, together with a rich theoretical understanding of the context and a strong research design, such large datasets can be immensely useful in guiding exploration and hypothesis generation. Just remain careful of Nicolas Cage movies.
I took a webinar on this topic on November 23, 2016. Click here to view the recording.